Current Projects
Computational Shape Analysis in Mitral Annular Disjunction
Mitral annular disjunction (MAD) is a cardiac abnormality that has been recently gaining attention, due its historical under-diagnosis and link with dangerous cardiac arrhythmia. MAD is characterized by a variation in the mitral valve’s attachment point. Usually the mitral valve is attached at the base of the left-ventricle, the hearts largest pumping chamber. In MAD the mitral valve is attached higher up than usual, partially in the left atrium. Recently, my colleagues at Oslo University Hospital linked MAD to an increased risk of cardiac arrhythmia, and suggested distance metrics to help identify the highest risk patients.
Why are patients with MAD at greater risk of dangerous cardiac arrhythmia? A leading hypothesis is that the higher position of the mitral valve puts additional stretch on the papillarly muscles, which are coupled to the mitral valve via the thin wire-like structures called the chordinea tendinea. The higher stretch could create spotaneous electrical activation waves, which could then destabilize the heart’s natural rhythm. My goal in this project is to look for signs of such mechanisms via 3D shape analysis of the left ventricle. Such analysis could yield important clues into why arrhythmias form in MAD patients, and which patients are at the highest risk.
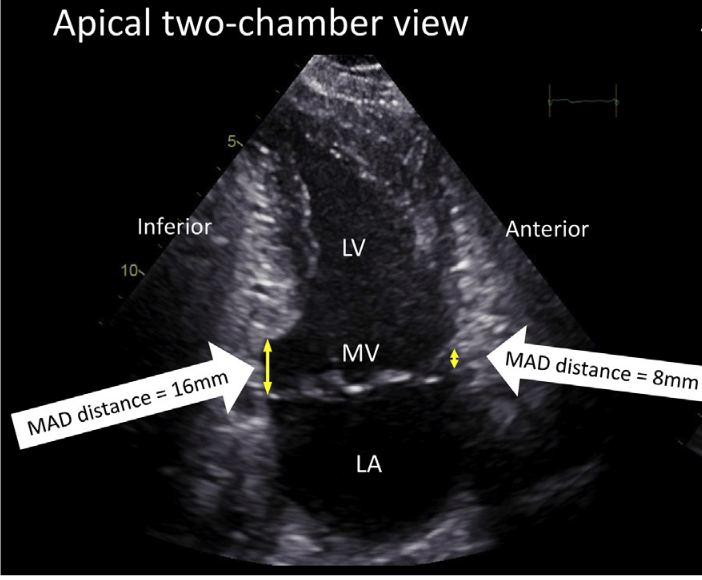
Special thanks to: My supervisor Molly Malleckar, cardiologist Eivind Westum Abel, Margareth Ribe, and ProCardio Centre Leader Kristina Haugaa.
Past Projects
T-Cell Receptor Sequencing: Computational Methods and Applications
Detecting and analyzing T-cell receptors is an exciting research area that is creating new diagnostic tests and medical technologies. This is because T-cells are an important part of your body’s immune system, and can kill cells that have been infected by a virus, and even attack cancers! However, under pathological situations, your body’s T-cells can attack healthy cells, and cause autoimmune disorders. Changes in your body’s T-cell population are therefore informative markers of disease and health, and understanding which T-cells are involved in which disease is a very active research area.
T-cells analysis is quite challenging though, as each T-cell is highly specialized and only reacts to a particular antigen, which it detects with receptors on the cell surface. Each person has somewhere between a million to a hundred million kinds of T-cell receptors in their body, so detecting a particular T-cell by its receptor can be very difficult. My main contribution to this problem is the development of a statistical methodology to quantify the probability of detecting a particular T-cell receptor with RNA sequencing of a blood sample. I also contributed to the first study in diagnosing Celiac disease based on T-cell receptors, and a comprehensive machine learning framework for immune receptor analysis.
Special thanks to: My supervisors Geir-Kjetil Sandve, Hedvig Nordeng, and collaborators Victor Greif, Shuo-Wang Qiao, Shiva Dahal Koirala, Ying Yao.
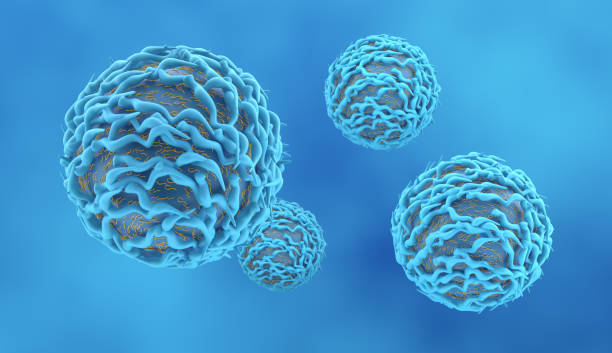
Quick and dirty scientific programming
How can computational scientists code quickly and efficiently, while maintaining a rigorous standard and bug free software? My collaborators and I tackled this challenging problem in our Ten simple rules for quick and dirty scientific programming. We developed our guide over the course of a pandemic struck 2021, when many of our scientific collaborations slowed down. We consequently had the time to pore over classical programming guides and hold lengthy zoom discussions. This process was definitely not easy, as programming habits are a kind of religion for many computer scientists, including me! In the end we agreed on 10 rules, which I believe are generally useful for anyone who wants to code efficiently in a scientific setting.
Special thanks to my discussion partners and coauthors: Geir-Kjetil Sandve, Ivar Grytten, Knut Dagestad Rand, and especially Lonneke Scheffer for being such a great artist and creating the cartoons! Thank you to the reviewers for challenging and useful feedback.
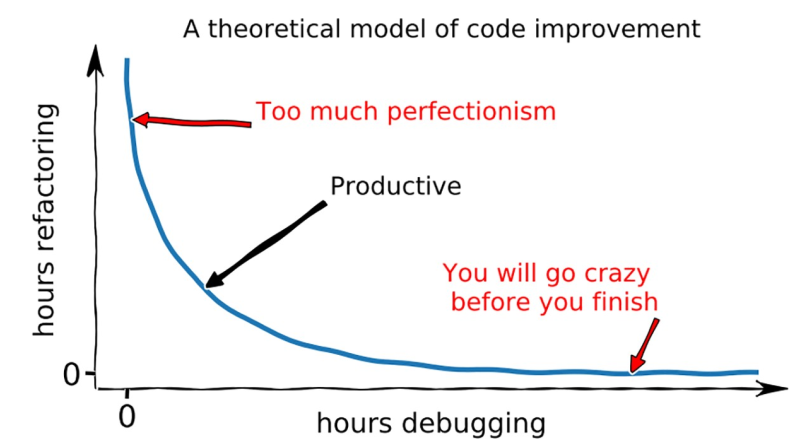
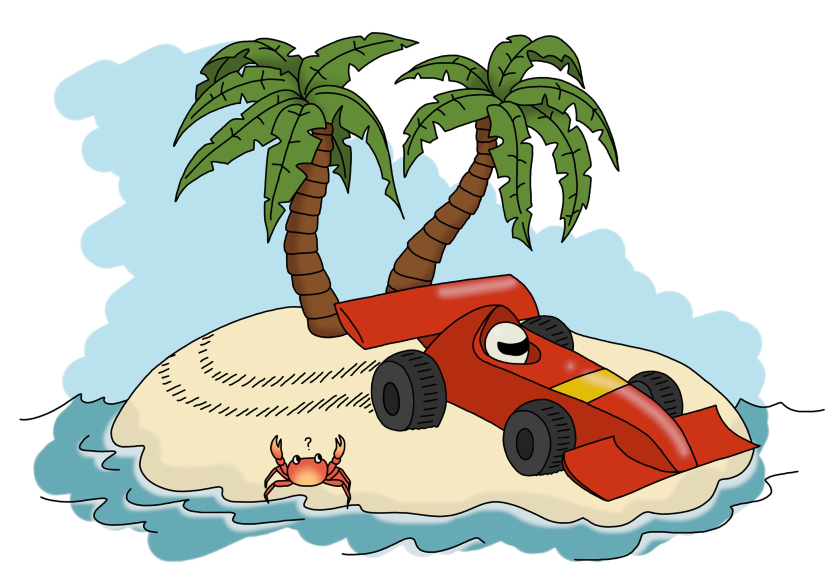
Computational Modelling and Prognosis of Sudden Cardiac Arrest in Non-ischemic Dilated Cardiomyopathy
From 2016-2019 I was a postdoctoral fellow at King’s College London, and had the opportunity to develop computer models of the electrical mechanisms that underlie sudden cardiac arrest. What an exciting but also emotionally charged topic! My father died of sudden cardiac arrest in 2007, and this has been motivating me ever since to create better prognostic models that can predict sudden cardiac arrest in advance. My hope is to allow for early treatment and prevention, or in the very least a chance for family members to spend quality time with a loved one before it is too late.
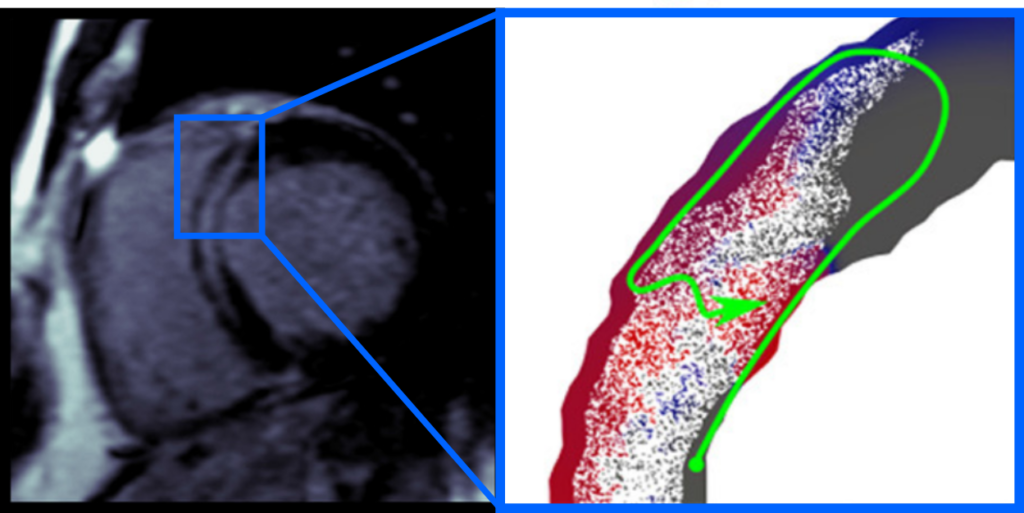
In London, my research was focused on the heart disease dilated cardiomyopathy, characterized by a balloon like shape and in many cases scarring in the heart. The non-ischemic variant of dilated cardiomyopathy, not caused by a lack of blood supply, is particularly mysterious as there are many potential causes, ranging from drug use to genetics. When I started my research there were well established methodologies for simulating the heart’s electrical activity in ischemic disease, but none for non-ischemic dilated cardiomyopathy. I therefore had the honour of pioneering this line of research together with my supervisor Martin Bishop. Together, Martin and I developed an MRI based electrical modelling technique for analyzing a patient’s heart scarring pattern, to see if it can initiate a pathological electrical activation pattern called a reentry. A reentry is essentially a short circuit, and is a crucial first step in a process of electrical breakdown which can stop the heart.
A key challenge that I faced in my research was the interpretation of the scars that I saw in my patient MRI images. Physical characteristics like the scar density and pattern are very important for properly simulating electrical reentries, but very little is known about them in dilated cardiomyopathy. In the end, I tested a wide variety of possible properties, which can cause a variety of different electrical reentry mechanisms. Then, when I tried correlating the simulation data to the patient outcomes, I noticed a very interesting trend. Patients who had more simulated electrical reentries tended to be at greater risk of sudden cardiac arrest! A very interesting finding which opens the door to using computer simulations to risk stratify dilated cardiomyopathy patients and select them for treatment.
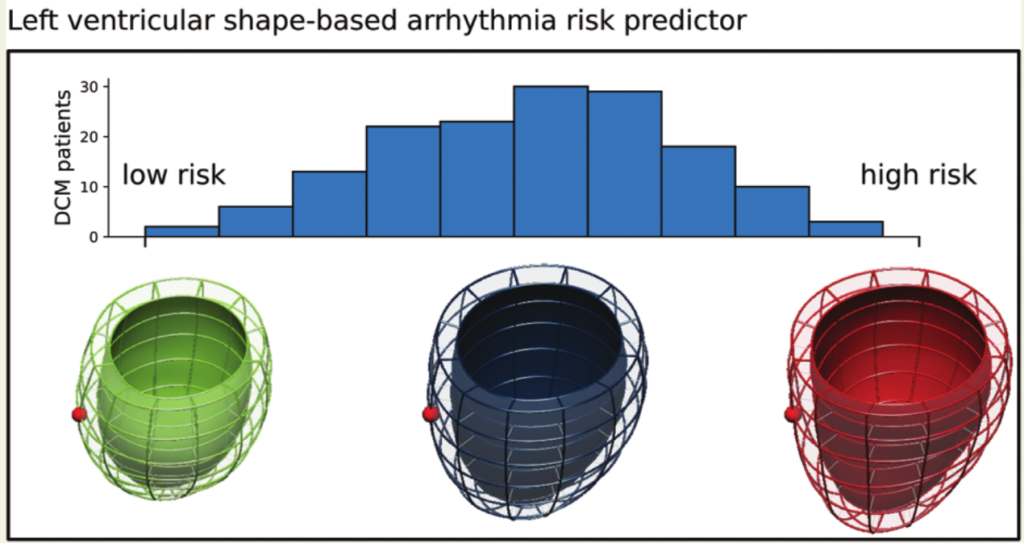
Parallel to the electrical simulations, I also developed a second approach to predicting sudden cardiac arrest in non-ischemic dilated cardiomyopathy. This approach was based on the analysis of the patient’s heart shapes, done in collaboration with shape modelling expert Pablo Lamata. Our idea was that early signs of electrical instability, such as tissue remodeling and or pathological ion channel dynamics, could potentially be detected by bulk shape changes to the left ventricle, the heart’s largest pumping chamber. Using a machine learning technique, Pablo and I analyzed the left ventricular shapes of 156 patients, and derived a pathological shape feature which was indicative of future sudden cardiac arrest. This finding, while exciting, needs to be validated in an independent cohort. If you are working with non-ischemic dilated cardiomyopathy and have MRI images and patient records of sudden cardiac arrest, please get in touch, I would be very interested in further testing and refining the shape model.
Special thanks to my supervisor Martin Bishop, and collaborators Sanjay Prasad, Brian Halliday, Pablo Lamata, Daniel Rueckert, Wenjia Bai, Caroline Mendonça Costa, and Christopher Aldo Rinaldi. Also thanks to my labmates from Steven Niederer’s group for being such good company and the many interesting scientific discussions. Finally, Thanks to my MEng student Charlotta Malvuccio for her work in MRI analysis.
High Dimensional Personalization of Cardiac Mechanics Models
Mechanical heterogeneities play a role in many cardiac diseases. For example, in coronary artery disease, an artery can become blocked, which results in a loss of blood supply to the tissues that are downstream from the block. The resulting damage is often localized to a part of the heart, which leads to an overall difference, or heterogeneity, in tissue properties. Damaged tissues often have worse contractility and are stiffer than healthy tissues. These tissue heterogeneities often play a large role in the subsequent development of the patient’s heart, as the heart attempts to compensate for the damage and provide enough blood to the body. Sometimes such compensatory mechanisms can lead to further damage. Sometimes they can lead to a partial recovery. Exactly what happens to any particular patient with damaged, highly heterogeneous, heart tissues is still a mystery. Though we have statistical trends, we lack mechanical certainty.
During my PhD, I developed new mechanical modelling techniques for estimating heart tissue heterogeneities from ultrasound motion data. I applied my techniques to a patient with cardiac dyssynchony, and also to a patient with myocardial infarction. The resulting personalized computational heart models were able to closely mimic the pathological motion patterns which we saw in the echocardiography images. These results are promising, and are being built upon by my colleagues at Simula. In the long term, I believe that we can gain novel insights into heart disease mechanisms, and eventually improve therapies and treatments.
Technologically, the key challenge that I dealt with was in finding an efficient optimization algorithm that could manipulate a virtual heart’s physical properties to match a patient’s heart motion. Such optimization problems become much harder when heterogeneities are considered, since the spatial differences in tissue properties means that more parameters need to be optimized. Many optimization methods suffer from the so-called curse of dimensionality, that is the amount of time they need to run grows exponentially with the number of extra parameters. Thankfully, my supervisor at the time, Marie Rognes, had developed some excellent software to efficiently solve such physical optimization problems, for which she won the 2015 Wilkinson prize for Numerical software. The application of this software and it’s mathematical method was the subject of my very first publication as a PhD student.
Special thanks to my supervisors Marie Rognes and Joakim Sundnes. Thanks as well to Simon Funke who helped with the optimization software that I relied upon, and Henrik Finsberg for being such a good research partner. Special thanks to the cardiologists at Rikshospitalet who helped me with the medical aspects of my thesis: Lars Dejgaard, Trine Håland, Stian Ross, and Einar Hopp.